- The Turing Point
- Posts
- The Turing Point - 30th Edition
The Turing Point - 30th Edition
For the best possible viewing experience, we recommend viewing this edition online.
đź“° Featured in This Edition:
Events
AI News Recap
Research Spotlight
đź—“ Upcoming: In AI Society
Neurons & Notions
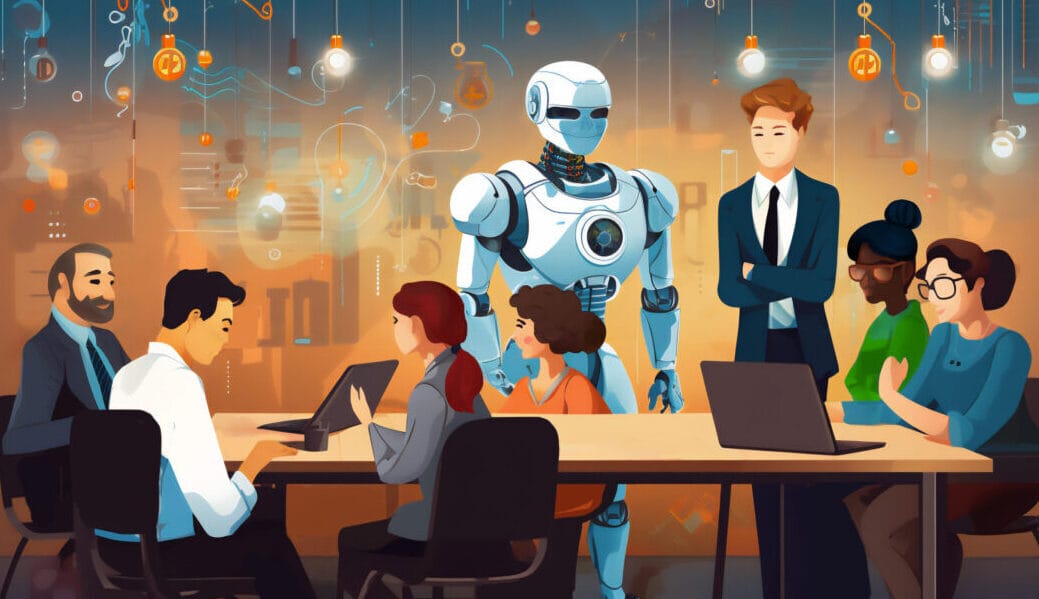
Image Credit: UGAResearch
Join AI Society for our fortnightly discussion sessions! Each session starts with 45 minutes of key AI news and trends from our newsletter, followed by 45 minutes exploring recent research papers and their potential impact. Stay informed, engage in discussions, and deepen your understanding of this rapidly evolving field.
We will also be uploading the discussion later on Youtube, so feel free to catch up with the session later!
đź“… Date: Wednesday Week 6, Term 1 (26/03/2025)
đź•’ Time: 1:00 - 2:30 pm
📍 Location: UNSW Business School 118
📺 Youtube Channel (Subscribe!): UNSW AISoc
MANUS AI: Revolutionising autonomy in modern AI
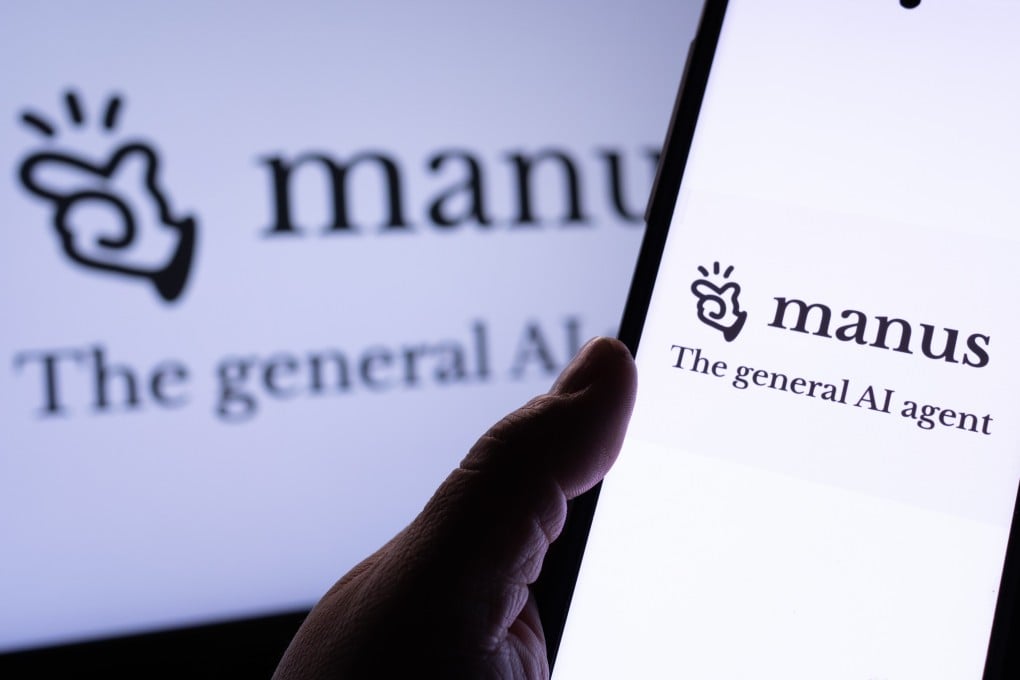
Although modern day LLM’s are capable of executing instructions and providing solutions in an efficient manner, what seems to differentiate MANUS AI from the rest of the market is it’s ability to independently do various tasks with minimal prompts. Each Manus session has it’s own individual computer, hence the way it processes information closely ties to how a human would execute these actions in a systematic yet creative manner.
The below example shows Manu AI in action, where the UI is split into two categories, one that represents Manus’s Computer and the other that represents the task to be achieved and it’s appropriate steps.

Key Features of Manus AI:
Autonomous Task Execution: One feature that sets Manus AI apart from the rest of the LLM’s is it’s ability to work asynchronously in the cloud, meaning an individual can go offline and be notified when the tasks are complete.
Multi-modal Task abilities: generates various data types such as text, images and code, while also seamlessly integrates with various tools such as web browsers, code IDE’s, and database management systems.
Adaptive Learning: Manus AI continually learns from user interactions and prompts, making each individual answer more tailored to user needs than ever.
These impressive features truly makes Manus AI lead the forefront of technological innovations, where it has outperformed other LLM’s by significant margins in the GAIA benchmark (test designed to evaluate AI’s problem solving capabilities in the real world).

GAIA is divided in 3 levels, where level 1 should be breakable by very good LLMs, and level 3 indicate a strong jump in model capabilities
Currently, Manus works on an invite only testing phase, and no pricing details have been released yet. Many have adopted to Manus’s power, strength, versatility and speed, however others have concerns over revealing an AI agent this advanced.
Published by Arundhathi Madhu, March 20 2025
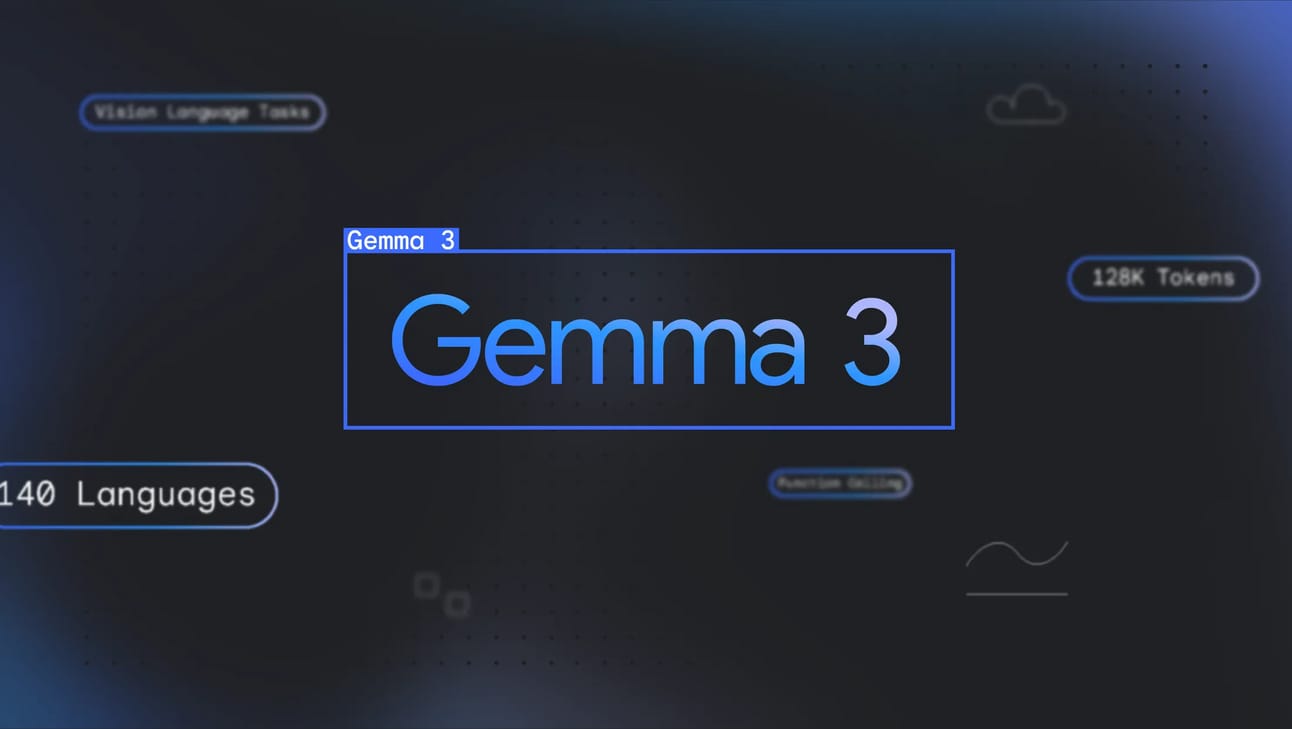
Gemma 3: Lightweight Power with State-of-the-Art Performance
Google has expanded its “Gemma-verse” with the launch of Gemma 3, a collection of lightweight, cutting-edge AI models built on the same foundation as its predecessor, Gemma 2. Designed for efficiency and portability, Gemma 3 is optimised to run directly on user devices—from smartphones and laptops to high-performance workstations.
The new release includes pre-trained models in multiple sizes—1B, 4B, 12B, and 27B parameters—allowing users to choose a model that best fits their hardware whilst enabling the scope for custom fine-tuning. While smaller AI models often come with trade-offs in quality, benchmark tests show that the 27B Gemma 3 outperforms DeepSeek-V3, demonstrating Google's advancements in balancing efficiency and capability.
Compared to Gemma 2, the latest iteration introduces key enhancements:
State-of-the-art performance within its size category, surpassing LLaMA 3-405B, DeepSeek-V3, and O3-Mini in preliminary human preference evaluations on LMArena’s leaderboard.
Expanded language support, now covering 35+ languages for broader accessibility.
Function calling capabilities, enabling more advanced AI-driven workflows.
A 128K-token context window, allowing the model to process and retain vast amounts of information in a single conversation.
With these upgrades, Gemma 3 sets a new benchmark for compact yet powerful AI models, making high-performance AI more accessible to a wider range of users and devices.
While Gemma 3 has been praised for its efficiency and accessibility, some concerns remain. Developers note that its openness is more restricted compared to fully open-source models like Mistral and LLaMA, limiting customisation. Additionally, while smaller versions run efficiently on local devices, the 27B model still demands high-end hardware, making that model less practical for casual users. Some skeptics also question its real-world performance beyond benchmarks, waiting for more extensive testing. With strong competition from models like GPT-4, LLaMA 3, and DeepSeek-V3, Gemma 3 will need to prove its long-term reliability and versatility to stand out.
Published by Aditya Shrivastava, March 20 2025

CSM-1B: A “sesame” That Changes The Conversational Speech Model
Sesame AI recently open-sourced its Conversational Speech Model (CSM), a speech generation tool capable of producing authentic-sounding audio using trained or custom voices.
Built upon Meta's robust Llama model, CSM-1B integrates an advanced audio decoder that employs residual vector quantization (RVQ). This enables CSM-1B to encode and recreate speech effectively, translating text and audio inputs into precise RVQ audio codes, thus enabling versatile and realistic voice synthesis.
Key technical highlights of the CSM-1B model include:
A multimodal architecture seamlessly integrating both text and audio inputs.
The ability to generate a wide variety of distinct voices without requiring specific fine-tuning.
Adoption of Mimi audio codes, allowing highly efficient compression at a mere 1.1kbps bitrate.
Capability to consistently maintain specific voice identities through acoustic "seed" samples.

Based on testing, CSM-1B performs exceptionally well on short phrases of 1-2 seconds, delivering impressively realistic audio outputs. However, as input length increases, the model’s output can occasionally become robotic or exhibit odd pauses, thus losing some coherence and experiencing slight voice distortion. Nevertheless, for a compact model containing only 1 billion parameters, this level of performance remains highly commendable. Notably, it can even be deployed effectively on personal computers with as little as 8GB of GPU memory.
Despite its technical capabilities, the open sourcing of CSM-1B also raises important ethical and safety questions. Combining voice cloning technology with a model like CSM-1B could have far-reaching and worrisome consequences. Imagine if someone could accurately clone your voice to credibly call a family member. How could the person answering the call be confident that they were talking to a real person and not an AI-generated voice? Our testing of CSM-1B showed that the conversations it generates are already very realistic, suggesting that we are not far from a time when the principle of "hearing is believing" will be overturned. While the creativity and productivity applications of this technology are exciting, the risks of abuse associated with it are equally great.
However, Sesame AI's vision goes far beyond creating advanced speech models. The company hopes to fundamentally transform the way humans and machines interact, making AI-generated speech more intuitive, natural, and engaging. By open sourcing CSM-1B, Sesame AI demonstrates its firm commitment to democratizing advanced speech technology, enabling developers and researchers around the world to improve, enhance, and responsibly deploy this powerful AI model.
Published by Dylan Du, March 20 2025

Image Credit: Google
Google’s Gemini Flash 2.0: A Leap Forward in AI Speed and Performance
Google has launched Gemini Flash 2.0, a major upgrade to its large language model (LLM) family, offering blazing speed and enhanced capabilities for a wide range of AI applications. Now generally available, it serves as a versatile "workhorse", designed to handle everyday tasks with increased efficiency.
Key Features of Gemini Flash 2.0
Speed and Efficiency: The model moves beyond its experimental phase, offering reliable AI solutions for businesses and developers. It provides faster response times compared to previous Gemini versions, making it ideal for real-time applications.
Multimodal Input: Gemini Flash 2.0 supports text, images, audio, and video, broadening its application scope.
1 Million Token Context Window: This feature allows for more comprehensive information processing, potentially eliminating the need for complex Retrieval-Augmented Generation (RAG) pipelines.
Native Tool Use: The model can interact with external tools and APIs, expanding its functionality beyond simple text generation.
Experimental Features: These include native image generation and a "Thinking" mode that enhances reasoning by generating thought processes.
Benchmarking Performance
Benchmark results show significant performance gains:
Gemini Flash 2.0 outperforms Gemini 1.5, with notable improvements in math, science, and multimodal reasoning tasks.
The Thinking Experimental model excels in reasoning-based tasks and operates twice as fast as Gemini 1.5 Pro.
New Offerings
Higher Rate Limits & Simplified Pricing: Flash 2.0 offers more accessible pricing and higher rate limits, making it more developer-friendly.
GitHub Copilot Integration: Enhanced accessibility for developers, allowing integration with tools like GitHub Copilot.
Simplified Document Processing: The large context window simplifies document processing, reducing the need for RAG pipelines.
A Step Toward the Future
Gemini Flash 2.0 is a significant leap in AI development, combining speed, performance, and multimodal capabilities. Google continues to refine the Gemini family, and models like Flash 2.0 are paving the way for faster, more efficient AI integration in everyday digital life.
Published by Shamim, March 20 2025

Image Credit: CLOXMEDIA
OpenAI’s Agents API
OpenAI's achievements in conversational artificial intelligence have earned it a reputation closely tied to ChatGPT, the chatbot we are so familiar with today. This innovation provided the company with a strong foothold in the AI industry, establishing it as the leader in natural language processing technologies. As the first model to effectively implement generative AI within a simple and versatile chatbot interface, ChatGPT experienced rapid growth in popularity following its release.
That being said, the arrival of new powerful players in the industry has intensified competition, making the AI landscape increasingly eager for further advancements within its existing scope. This chaotic environment also highlighted ChatGPT’s limitations — a side effect of its versatility.
In an official survey conducted by OpenAI, ChatGPT users shared their experiences with the tool. A common concern was the difficulty in obtaining production-ready responses for complex multi-tool task execution. This challenge arises from ChatGPT’s sole reliance on prompt input to generate appropriate responses — a characteristic that was once the product’s defining strength. In a complex environment, the limitations of natural languages, such as ambiguity and limited scalability, are amplified, making it increasingly difficult to interpret inputs accurately. This often results in reduced system performance and inefficiencies in task execution.
The OpenAI Agents API emerges as a direct response to these challenges. Designed to enhance ChatGPT’s capabilities, this API introduces a powerful framework for integrating external tools and allowing AI systems to perform complex tasks autonomously. Unlike ChatGPT, which solely relies on natural language prompts, the OpenAI Agents API enables systems to dynamically interact with various tools and APIs, making decisions and executing steps without requiring constant user guidance.
By allowing the use of multiple specialized tools in a coordinated manner, the Agents API addresses the limitations inherent to natural language processing. It transforms a traditionally passive system into an active problem-solving entity capable of performing intricate tasks such as data retrieval, file processing, statistical analysis, and more. The result is a system that can efficiently interpret and fulfill complex requests, providing more reliable and production-ready outputs.
Published by Victor, March 20 2025
Stargate: Tump’s $500 Billion AI Initiative

Image Credit: BBC News
On only the second day of his new term in office, US President Donald Trump announced a staggering $500 billion initiative for AI development, infrastructure, and innovation named Stargate. Stargate is a private joint venture that aims to build 20 large AI datacenters in the US, with an initial investment of $100 billion and scaling up to $500 billion total by 2029. This is a landmark move from the US government as they dedicate such staggering resources to no doubt position the country as the global leader in artificial intelligence, amidst fierce competition from international rivals like China. This initiative also stands uncontested in terms of scale, as made evident when compared to previous US government projects:
Manhattan Project: Roughly $35 billion dollars (adjusted for inflation) [~1.5% of US GDP in the mid-1940s]
Apollo Program: Roughly $170–$180 billion (adjusted for inflation) [~0.5% of US GDP in the mid-1960s]
Space Shuttle Program: Roughly $275–$300 billion (adjusted for inflation) [~0.2% of US GDP in the early 1980s]
Stargate as mentioned is $500 billion dollars, which amounts to roughly 1.7% of the US GDP in 2024, stating AI’s importance to future global and economic landscape. In tune with this, in their statement OpenAI says that “This infrastructure will secure American leadership in AI, create hundreds of thousands of American jobs, and generate massive economic benefit for the entire world.”
Joining Trump for the announcement were key figures in AI and technology including Sam Altman (CEO of OpenAI), Larry Ellison (chairman of cloud giant Oracle) and Masayoshi Son (CEO of Japanese giant SoftBank). These three companies along with the Abu Dhabi government’s AI focused investment fund MGX form the principals of the joint venture. Microsoft, Nvidia and Arm also join as key contributors
In terms of structure, SoftBank handles financial responsibilities, with CEO Masayoshi Son also serving as Chairman, and OpenAI has operational responsibility which includes the development and training of AI models. Since AI is inherently reliant on massive amounts of high-quality and readily accessible data, Oracle will use its data-handling expertise to source these Stargate datacenters. This however, is only a high level breakdown of the venture’s organisation, with finer details not finalised or made public just yet. Regardless, the project has already gone full steam ahead as an initial buildout of 10 data centers, 46,000 square meters each and equipped with nearly hundred thousand Nvidia GPUs has already begun.
Although the project promises an AI focused industrialisation, how that will manifest in terms of use cases such as a widely available AI cloud, enterprise level development platforms, AI hosting and GPU services, etc, is still up in the air. Regardless, such a commitment over the next 5 years, is sure to lead to monumental developments in AI progress which could promise benefits in science, research, incredible new products and services and if OpenAI is successful, even AGI.
Published by Abhishek Moramganti, March 20 2025
Phi-4: Microsoft’s Small But Mighty AI Model

Image Credit: Hiverlab
Microsoft’s Phi-4 series is making waves in the AI world, offering powerful language and multimodal capabilities in a compact, efficient package. Unlike massive AI models that require extensive computing power, Phi-4 delivers high performance without heavy resource demands, making it a practical choice for developers
The Phi-4 series comes in two versions:
Phi-4 Mini – Focused on text-based tasks like writing, summarization, and code generation.
Phi-4 Multimodal – Designed to handle text, images, and audio, making it great for things like visual question answering and speech recognition
One of Phi-4’s biggest strengths is its ability to perform well while staying lightweight. It uses a sparse attention mechanism, which makes processing faster and more efficient without sacrificing accuracy. Developers can also fine-tune it easily using methods like LoRA (Low-Rank Adaptation) and prompt tuning, so it can be customized for different applications without needing to be retrained from scratch.
When it comes to benchmarks, Phi-4 holds its own against larger models. It outperforms competitors in tasks like document understanding, speech translation, and even complex reasoning with charts and tables. Despite being smaller than many leading AI models, it remains just as (if not more) effective in real-world applications. Best of all, Phi-4 is open-source, meaning developers have access to pre-trained models, fine-tuning tools, and documentation to get started quickly. Setting it up is simple, and it integrates easily into existing workflows.
Phi-4 is proof that bigger isn’t always better. It’s a versatile, efficient AI model that’s accessible to more developers without compromising on performance. Whether you’re working with text, images, or speech, Phi-4 is a solid option for building smarter applications with less computational overhead.
Published by Harika Dhanisiri, March 20 2025
đź“‘ Research Spotlightđź’ˇ
The New Reasoning Era In The Latent Space
February 2025
Closing Notes
As always, we welcome any and all feedback/suggestions for future topics here or email us at [email protected]
Stay curious,

🥫Sauces 🥫
Here, you can find all sources used in constructing this edition of Turing Point:
Phi 4:
https://hiverlab.com/microsofts-phi-4-a-new-era-of-ai-models/
https://sebastian-petrus.medium.com/phi-4-c634daad886b#:~:text=What%20is%20Phi%2D4%3
Stargate:
https://www.abc.net.au/news/2025-01-22/stargate-ai-explained/104846290
CSM 1B:
https://www.rdworldonline.com/a-quick-demo-of-sesame-ais-open-source-conversational-speech-model/
https://the-decoder.com/sesame-releases-csm-1b-ai-voice-generator-as-open-source/
https://www.arun.blog/sesame-voice-demo/
https://community.modelscope.cn/67d904d43b685529b70d6e4e.html
Gemma 3:
https://blog.google/technology/developers/gemma-3/
Gemini Flash 2.0:
https://www.datacamp.com/blog/gemini-2-0-flash-experimental
https://developers.googleblog.com/en/gemini-2-family-expands/
OpenAI Agents API
For the best possible viewing experience, we recommend viewing this edition online.